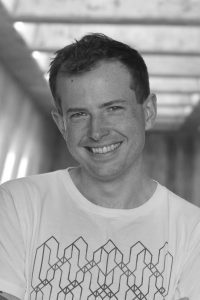
The Canadian Association for Neuroscience is proud to announce that Dr. Blake Richards, from University of Toronto at Scarborough, is the winner of the 2019 CAN Young Investigator Award. This award recognizes his outstanding research achievements at the intersection of neuroscience and Artificial Intelligence (AI). Dr. Richards will receive this prize on May 24, 2019 in Toronto, during the 13th Annual Canadian Neuroscience Meeting.
“Dr. Blake Richards’ work provides an interdisciplinary perspective, mixing theory and experiments to a degree that is truly rare, despite its importance for moving neuroscience forward in the coming decades. […] I am confident that he will continue his upward trajectory and emerge as one of the world’s leaders in computational techniques for understanding the brain.”
Melanie A. Woodin
Professor, Department of Cell and System Biology
Vice-Dean, Interdivisional Partnerships – Faculty of Arts and Science
University of Toronto
Dr. Richards’ research program focuses on neural computation, learning, and artificial intelligence (AI). Using a combination of computational modelling and advanced neuroscience and brain imaging approaches, his lab is exploring the neural basis of deep learning. The goal of this work is to better understand the neurobiological basis of animal and human intelligence and provide new insights to help guide AI development.
His laboratory has made several important contributions to mathematical models of learning and memory in the brain. These have provided new insights on the process of memory consolidation, learning in the brain and by machines, and how brain structures permit deep learning in real brains. This theoretical work has been well-recognized in both the neuroscience and AI communities, and Dr. Richards is considered a leading researcher at this disciplinary intersection. AI is currently being revolutionized with brain-inspired mechanisms.
“Despite all the progress in AI, the real brain is still the most sophisticated learning device on Earth, and no AI can yet match the general-purpose intelligence of humans. At the same time, AI can help us to revolutionize our understanding of the brain, providing means for analyzing and interpreting previously uninterpretable aspects of the biological basis of intelligence. Dr. Richards is uniquely well poised to conduct research at the interface of neuroscience and AI, thanks to his background as both a computer scientist and an experimental neuroscientist. Very few researchers in this world possess his ability to speak the languages of both machine learning and neuroscience so fluently.”
Yoshua Bengio
Scientific Director of the Mila, Quebec Artificial Intelligence Institute
Full Professor, Department of Computer Science and Operations Research,
Université de Montréal
.
“His program of research positions him between the disciplines of AI, neurophysiology and behavioral neuroscience. It is in these spaces – the gaps between traditional research disciplines – that there is enormous scientific opportunity. Blake ably straddles these multiples fields, and he is already exploiting the riches of such an advantageous position.”
Paul Frankland, Senior Scientist
Canada Research Chair in Cognitive Neurobiology
Professor, Departments of Psychology, Physiology and Institute of Medical Science
University of Toronto
Dr. Richards has received several awards and recognitions for his contributions. In 2016 he was awarded a Google Faculty Research award for his research on memory and reinforcement learning; in 2017 he became a Fellow of the CIFAR (Canadian Institute for Advanced Research) Learning in Machines and Brains Program; in 2018 he received an Early Career Researcher Award from the Government of Ontario; and most recently he was nominated as a Faculty Affiliate to the Vector Institute for AI. These recognitions are in addition to the funding he has received for his research from several highly competitive sources, including the Canada Foundation for Innovation, the Natural Sciences and Engineering Research Council of Canada, the Human Frontier Science Program, the Allen Institute for Brain Science, and Google. Most recently, Dr. Richards was awarded one of 29 Canada CIFAR AI Chairs as part of the Pan-Canadian AI Strategy.
In addition to his research contributions, Dr. Richards has been an active member of the neuroscience and AI communities. Together with Dr. Timothy Lillicrap from Google DeepMind, he organized a workshop on deep learning and neuroscience at the 2016 Computational and Systems Neuroscience Conference (COSYNE). He also co-organized a Canadian Institute for Advanced Research (CIFAR) Brain Symposium last year, which brought together neuroscientists and machine learning experts, and which has sparked several new, interdisciplinary collaborations in the Canadian research community. And, more recently, he helped to organize a breakout session on memory consolidation at the 2018 Cognitive and Computational Neuroscience Conference in Philadelphia. Finally, Dr. Richards is recognized, by all who have worked with him in a laboratory, as a natural leader who truly enjoys mentorship.
Dr. Richards has shared his discoveries outside the scientific community, through numerous interviews to the popular press (including The New York Times, The Independent, The BBC, and NPR), and speaking arrangements at public events such as Pint of Science and NeuroTechX. He is always engaging and easy to understand in his public appearances and can act as a great ambassador for research into the links between AI and neuroscience. He has graciously accepted to host the 2019 CAN Public lecture with Geoffrey Hinton on May 21, 2019 in Toronto.
Dr. Blake Richards is an exceptional young investigator, whose work seamlessly integrates advanced neuroscience, neuroimaging, computational and artificial intelligence approaches to advance our understanding of the brain, but also to contribute to the development of artificial intelligence. The Canadian Association for Neuroscience is very proud to name him the 2019 CAN Young Investigator.
Visit the Learning in Neural Circuits (LiNC) Laboratory website: linclab.org
Five Most Significant Published Papers
- J Guergiuev, TP Lillcrap and BA Richards (2017) Towards deep learning with segregated dendrites. eLife, 6: e22901 https://elifesciences.org/articles/22901
This paper was one of the first ever computational models to demonstrate how the real brain could potentially implement a deep learning style credit assignment algorithm. It utilized a conductance-based model of spiking, three-compartment pyramidal neurons arranged in a multilayer structure. It demonstrated that, thanks to the segregation of feedforward sensory signals and higher-order feedback in different dendritic compartments of pyramidal neurons, neuron-by-neuron credit assignment could be achieved and images could be classified with near-human accuracy. This opened the door to linking deep learning theory from artificial intelligence with neurobiology.
- BA Richards and PW Frankland (2017) The persistence, and transience, of memory. Neuron, 94 (6): 1071-1084. https://www.sciencedirect.com/science/article/pii/S0896627317303653
This was a review paper, but it has had a large impact on the field. It provided researchers with a new framework for thinking about forgetting. It reviewed existing literature on the neurobiology of forgetting and showed that the evidence supports the conclusion that forgetting is an active process induced by a number of cellular mechanisms, not merely a failure of the brain’s mnemonic systems. Theory from artificial intelligence was used to put forward proposals for why forgetting is a potentially beneficial thing, in moderation. Specifically, neural networks research shows that forgetting can improve behavioural flexibility and generalization. This provides neurobiologists studying memory with a new way of thinking about forgetting in healthy brains.
- BA Richards, F Xia, A Santoro, J Husse, MA Woodin SA Josselyn, and PW Frankland (2014) Patterns across multiple memories are identified over time. Nature Neuroscience, 17 (7): 981-986. https://www.nature.com/articles/nn.3736
Ever since David Marr’s seminal work in computational neuroscience, it has been postulated that memory consolidation serves to identify statistical patterns present in multiple memories.
However, until this paper, Marr’s idea had not been tested experimentally. Dr. Richards developed a version of the Morris water-maze where mice develop multiple memories for platform locations, such that the locations contain a hidden statistical pattern. He also developed a software package for performing information theoretic measurements on watermaze data that can measure the extent to which a mouse’s swim path matches a statistical pattern. Using these tools, they demonstrated that memory consolidation does indeed promote the identification of patterns across multiple memories. This confirmed a long-standing, but untested, hypothesis about memory consolidation in computational neuroscience.
- AM Muldal, TP Lillicrap, BA Richards and CJ Akerman (2014) Clonal relationships impact neuronal tuning within a phylogenetically ancient vertebrate brain structure. Current Biology, 24 (16): 1929-1933. https://doi.org/10.1016/j.cub.2014.07.015
This paper combined single-cell labelling and in vivo 2-photon Ca2+ imaging to demonstrate that neurons in the midbrain that derive from the same neural progenitor cells have similar receptive fields. This was a major finding, linking cell lineage to computation. Dr. Richards designed and built the in vivo 2-photon imaging system used in this work and collected the preliminary data.
- BA Richards, OP Voss and CJ Akerman (2010) GABAergic circuits control stimulusinstructed receptive field development in the optic tectum. Nature Neuroscience, 13 (9): 1098-1106. https://www.nature.com/articles/nn.2612
In this paper, which came out of Dr. Richards’s work at the University of Oxford, he discovered a novel role for inhibitory circuits in promoting visual learning. Using a bespoke system that Dr. Richards had developed for in vivo patch clamping, he showed that inhibition in the developing brain removes correlations in electrical activity that overwhelm synaptic plasticity mechanisms. These findings have helped to understand how disrupted inhibitory signalling can affect brain maturation, and opened the door to later studies looking at the role of inhibition in shaping neural plasticity (see Van Rheede, Richards and Akerman (2015), Neuron, 87 (5): 1050-1062).
Media coverage
Wall Street Journal article on adaptive forgetting: https://tinyurl.com/ycfzmwdh
Forbes article on CIFAR summer school: https://tinyurl.com/ycj64nz9
Article on Singularity Hub on our recent eLife paper: https://tinyurl.com/y8o7k24m
CIFAR announcement on recent eLife paper: https://tinyurl.com/y7frag93
Interview with The New York Times on the role of forgetting in learning: https://tinyurl.com/y9m8ntw4
Interview with The Times on forgetting: https://tinyurl.com/y7ax8x24
Interview with NPR on the importance of forgetting for intelligence: https://tinyurl.com/y9xlk3gq
Coverage of forgetting article on CNN: http://tinyurl.com/y7puhog8
Coverage of forgetting article at The Independent: http://tinyurl.com/yb9rhh3b
Interviews with The Verge on brain-machine interfaces: https://tinyurl.com/musmf55 and https://tinyurl.com/lkyjzd3
Young Investigator award and talk – May 24 5:30PM
Sponsored by the Montreal Neurological Institute
Credit assignment via spike-based causal inference
Learning in neural circuits requires a means of assigning “credit” to each neuron for its contribution to behaviour. In hierarchical circuits, like the neocortex, credit assignment is challenging, because a neuron’s contribution to behaviour depends on its impact on downstream circuits, which may involve multiple synaptic connections and pathways. One way of understanding this challenge is in analogy to the causal inference question faced by many researchers: how can we determine the causal impact of one variable on another when there are many potential interactions and many uncontrolled variables? In this talk, I will discuss modelling work from my group that was inspired by causal inference tools from economics. These tools use discontinuities in a variable to infer causal relationships, even when most variables in the system cannot be controlled for. I will show how the discontinuity introduced by action potentials can be used by neurons to estimate their causal impact on downstream circuits. Furthermore, I will show how this could help neurons to solve the credit assignment problem. I will end with a discussion of the predictions generated by this model of learning in the brain, in order to provide experimentalists with a means of testing these ideas.